Speaker Details
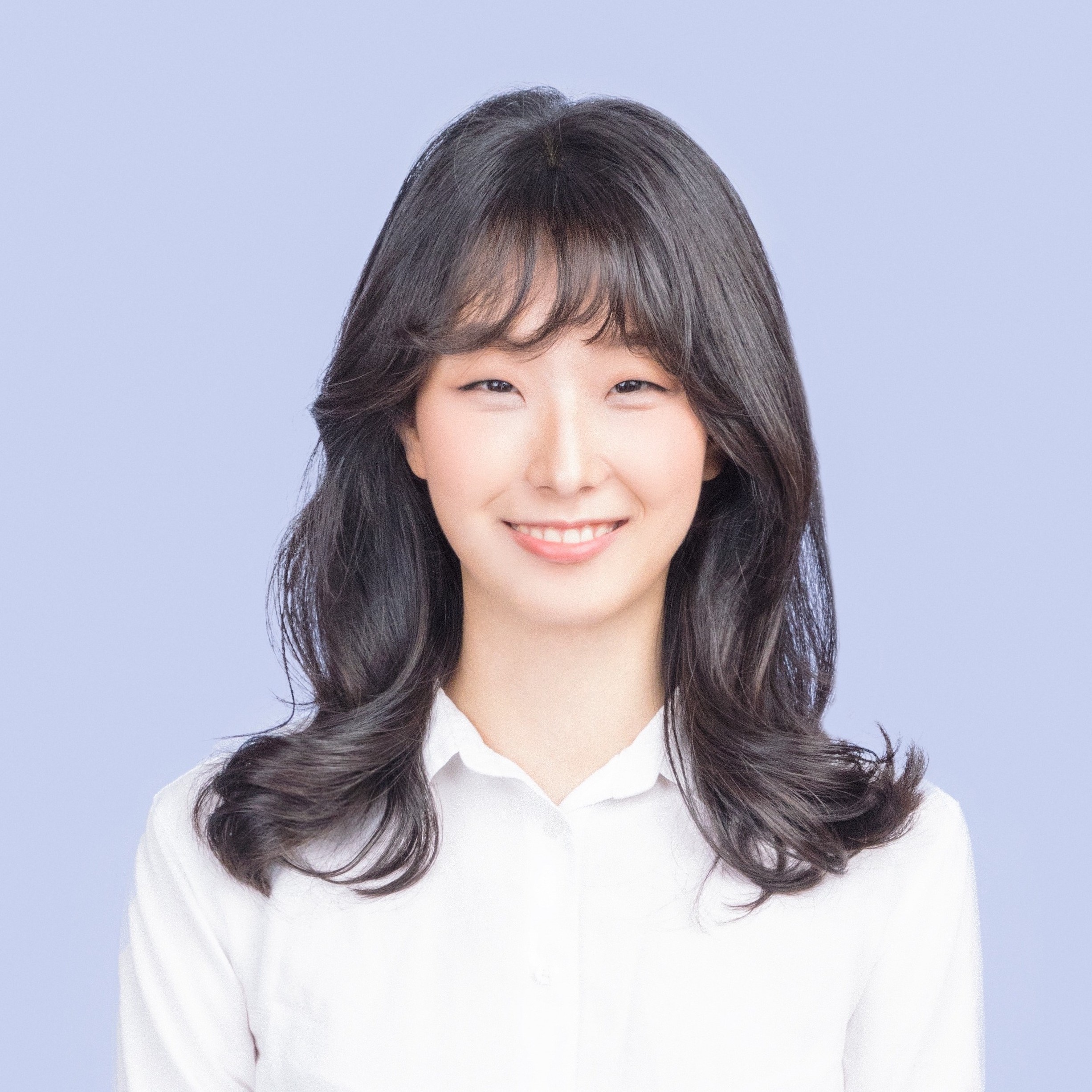
Sewon Min
University of Washington
Sewon Min is an incoming assistant professor at UC Berkeley EECS. She recently received her Ph.D. in Computer Science & Engineering from the University of Washington. Her research focuses on language models (LMs): studying the science of LMs, and designing new model classes and learning methods that make LMs more performant and flexible. She also studies LMs in information-seeking, legal, and privacy contexts.
She won a paper award at ACL 2023, received a J.P. Morgan Fellowship, and was named an EECS rising star in 2022.
Previously, she was a part-time visiting researcher at Meta AI, interned at Google and Salesforce, and earned her B.S. in Computer Science & Engineering from Seoul National University.
Talk
Title: Distributed Language Models: Isolating Data Risks
Abstract: The impressive capabilities of large language models (LMs) are derived from their training data, which is often sourced from the web. However, these datasets also present issues concerning copyright and the lack of consent from data owners. Understanding the degree to which LMs depend on this data, and developing models that adhere to their restriction, remain significant challenges. In this talk, I will first introduce the Open License Corpus (OLC), a new corpus comprising 228 billion tokens of public domain and permissively licensed text. Despite its vast size, models trained solely on the OLC experience performance degradation due to limited domain coverage. We then propose SILO, a new language model that combines a parametric LM with a modifiable nonparametric datastore. This datastore, containing copyrighted books and news, is only accessed during inference. This methodology allows for the use of copyrighted data without directly training on it, enables sentence-level attribution of model outputs to data sources, and provides an opt-out mechanism for data owners. Finally, I will outline our roadmap towards “distributed models”, a set of model components that use data with varying levels of restrictions—such as public domain, copyrighted, or unreleasable data—in different capacities, such as for training, as a datastore, or in other ways.